7 Principles to Guide Responsible Development of AI Systems
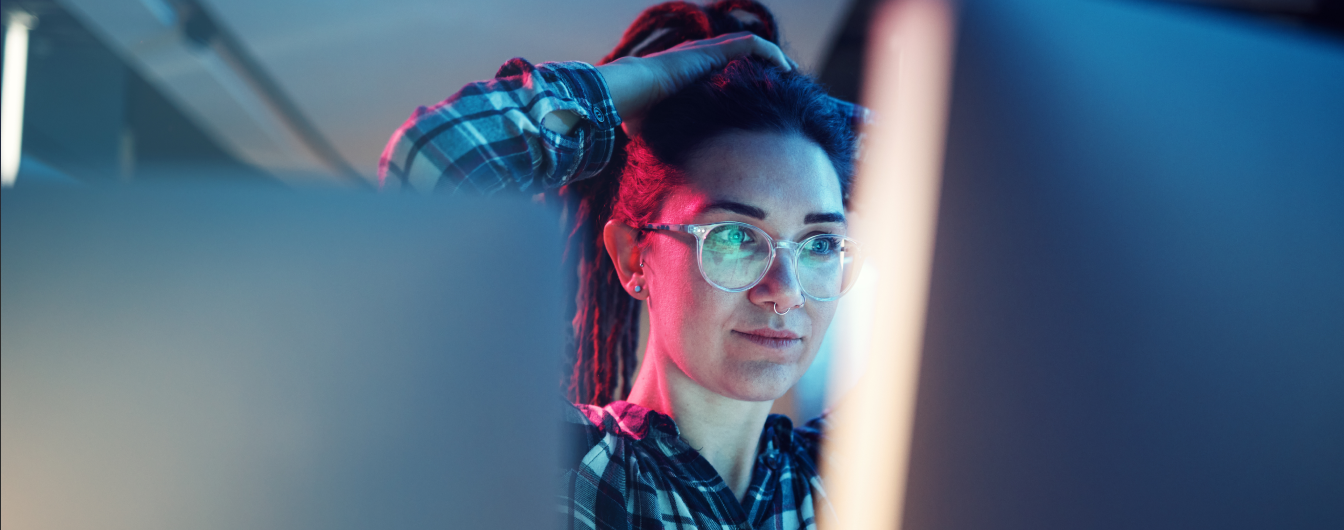
There’s immense opportunity in developing AI and machine learning technologies that foster and grow individual and collective wellbeing for humankind. Just as AI presents the potential to benefit all people, so too does it present growing risks due to our increased adoption and dependence, wittingly and unwittingly, on AI-powered systems. To reach that potential and minimize and mitigate risks, we believe that AI development and deployment should be guided by several principles.* These responsible AI principles include:
- Human-Centered Design: AI systems should be designed with the needs and values of humans in mind. In fact, human wellbeing should be prioritized over other considerations. Societal and individual outcomes should be paramount in both design and evaluation, defining the scope of operation for all other functional and operational characteristics. These solutions must continuously be monitored as they are deployed and continue to change over time to ensure that this principle is durable across the lifecycle.
- Awareness: Everyone who works on AI technologies must be aware of the context and impact of their actions and decisions. Understanding the details of the AI system you are working on is key, including the problem you are trying to solve and the consequences of the AI recommendations. The risks to both direct users of the technology and implications for wider social impact of these technologies should always be considered.
- Data Understanding, Privacy & Security: It’s important that everyone who works on AI technologies has a deep understanding of the data, including genealogy and provenance, they are working with and the way it is used. System design and implementation choices should align with relevant regulatory and legal practices. A proactive approach to data protection needs to be adopted to constantly evaluate potential vulnerabilities, risks and biases pertaining to both system operation and the minimization of data breaches or unauthorized access to sensitive information. Respecting individuals’ privacy and data protection rights should be a governing principal of system design and development activities.
- Fairness & Non-Discrimination: AI systems must be designed and deployed in a way that avoids discrimination and bias, and that promotes fairness and equality. This involves everyone who works on AI technologies to ensure the system being set up is fair, unbiased and does not perpetuate or exacerbate existing inequalities. AI development teams should integrate diverse and multidisciplinary perspectives to help identify these risks.
- Social & Environmental Responsibility: It’s important to consider the social and environmental impact of AI systems and strive to minimize any negative impact. Analyze the system’s impact, take steps to mitigate any negative impact and consider the benefit the system provides commiserate with the costs and risks of its operation. One option is for an independent team to evaluate these impacts.
- Repeatability & Testing: AI systems should be designed, tested and deployed in a way that ensures that their performance and behavior can be replicated and verified. This includes assessing and understanding the variability in behavior inherent to some systems and the true performance of algorithmic systems, including human variability. Implement a standard of testing prior to production that includes tests for all principles outlined here. Testing should also be done during development and a plan for continuous monitoring and evaluation should be enacted. Design and deployment should be improved over time to maximize the positive impact on users and constantly monitor for any negative impacts or changes in expected or observed performance.
- Accountability: Every individual working on AI technologies needs to acknowledge a shared responsibility for the impact of their system(s) on individuals and society. One person or a group of people for every system component should be held accountable for the development and communication of risks and intention-driven decisions. The development team should uphold auditing standards and an accountability chain. It’s important to design and develop a monitoring and mitigation plan for any harms originating from the AI technology.
Responsible AI doesn’t put humans in the loop as a regulatory compliance measure; it puts humans at the center of the system design process because the goals, outcomes, behaviors and performance (including measurement) are fundamentally about human wellbeing. Responsible AI means putting human wellbeing at the center of all system design choices in a considered, intentional and systematic manner… everything else is opportunistic AI.
Interested in learning how your AI systems, or hypothetical use cases, stand up to regulatory and technical risk? Take our free assessment today!
GPT-4 was leveraged to partially generate this blog.
This article was originally published on epam.com/insights. The article’s main photo is from envato.com.
The authors of this article are:
- Martin Lopatka. Director, Data Analytics Consulting, EPAM.
- Aleksandr Chikovani. Chief Systems Engineer, EPAM.
- Eric McVittie. Manager, Data Analytics Consulting, EPAM.
- Nora Skjerdal. Consultant, Legal & ERM Consulting, EPAM Continuum.
- Kathryn Hughes. Principal, Legal & ERM Consulting, EPAM Continuum.
Podobne artykuły
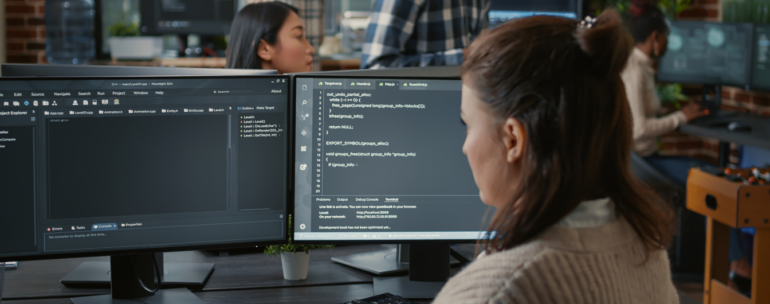
Data Security in an AI-First Paradigm
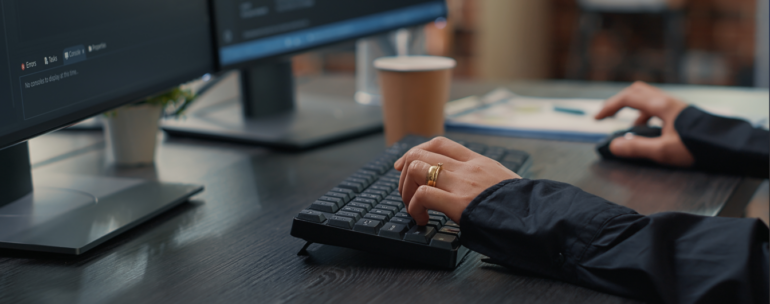
AI-Accelerated Innovation: The Next Evolution of Software Engineering
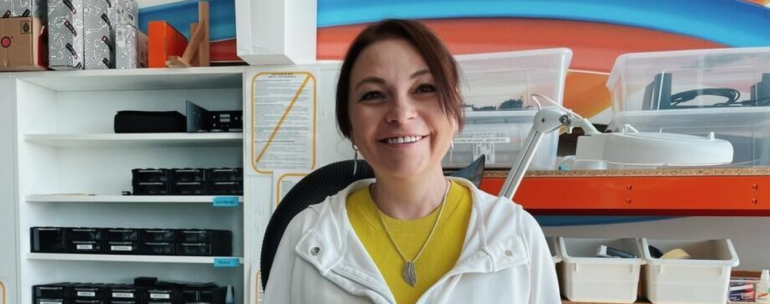
EPAM Team in the Final for NASAs MarsXR2 Challenge. Interview with Nataliia Savchenko
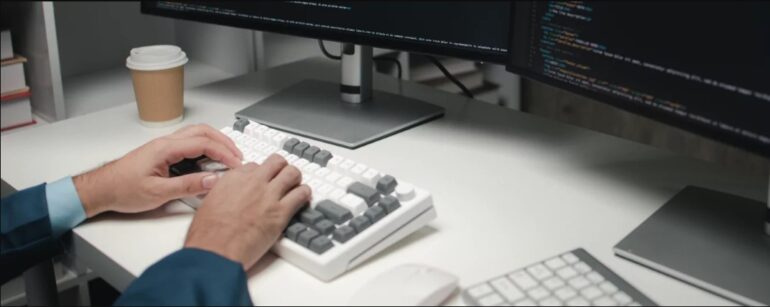